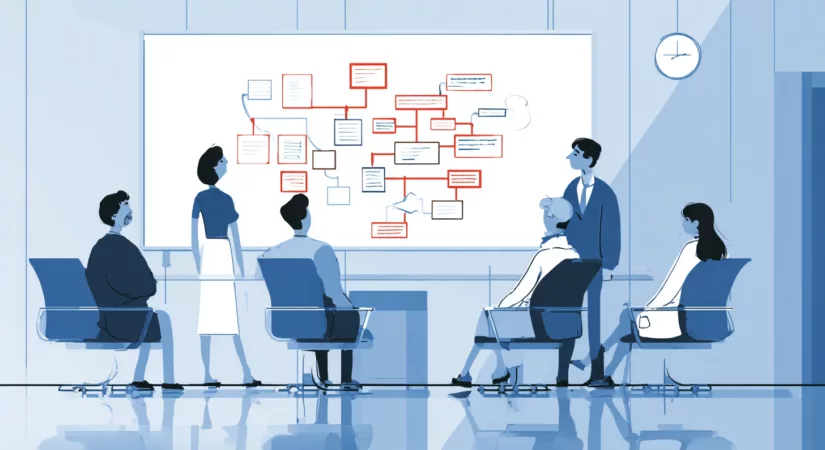
Frame It to Win - Action Plan Template
Download this action plan template to plot out your 90-day roadmap and structure your business context, charter, operating model, and delivery plan.
Explore insights from IIA and select content from our research library below. Our content is always independent and driven by our clients’ everyday problems, challenges and initiatives.
Download this action plan template to plot out your 90-day roadmap and structure your business context, charter, operating model, and delivery plan.
Our latest webinar uncovering what it's like inside an organization undergoing a transition to a federated model featuring Keith Moody, vice president of analytics at Discount Tire
Use this practical guide to explore how a federated operating model can help complex enterprises balance autonomy with alignment, unlocking sustainable, enterprise-wide impact.
Use this high-level checklist to surface readiness signals. Count how many items you can confidently check.
What challenges are you facing in your analytics operating model? In this executive summary from an exclusive, invite-only discussion, D&A leaders cutting across industries discuss their approach to federated analytics.…
Building a data strategy that is aligned with the business expectations of stakeholders is key to delivering a strong return on analytics investments. Our experts put together the insights for…
Learn how a federated analytics operating model helps you show value early and often while building your data strategy. Get the starting blueprint to transition to—or reset—federated analytics.
Join Ryan Sousa, IIA Expert and former CDAO at Seattle Children’s Hospital, to explore the virtuous learning cycle of data fluency for continuous enterprise growth and innovation.
Research & Advisory Network clients have full access to our research library including strategic frameworks, topic deep dives, leading practices from blue-chip companies and brief responses on the most common topics being worked on across the client community. In addition, clients can directly engage with authors through IIA’s Expert Network.
Looking for more? Contact us today about how we can connect you to our Expert Network.